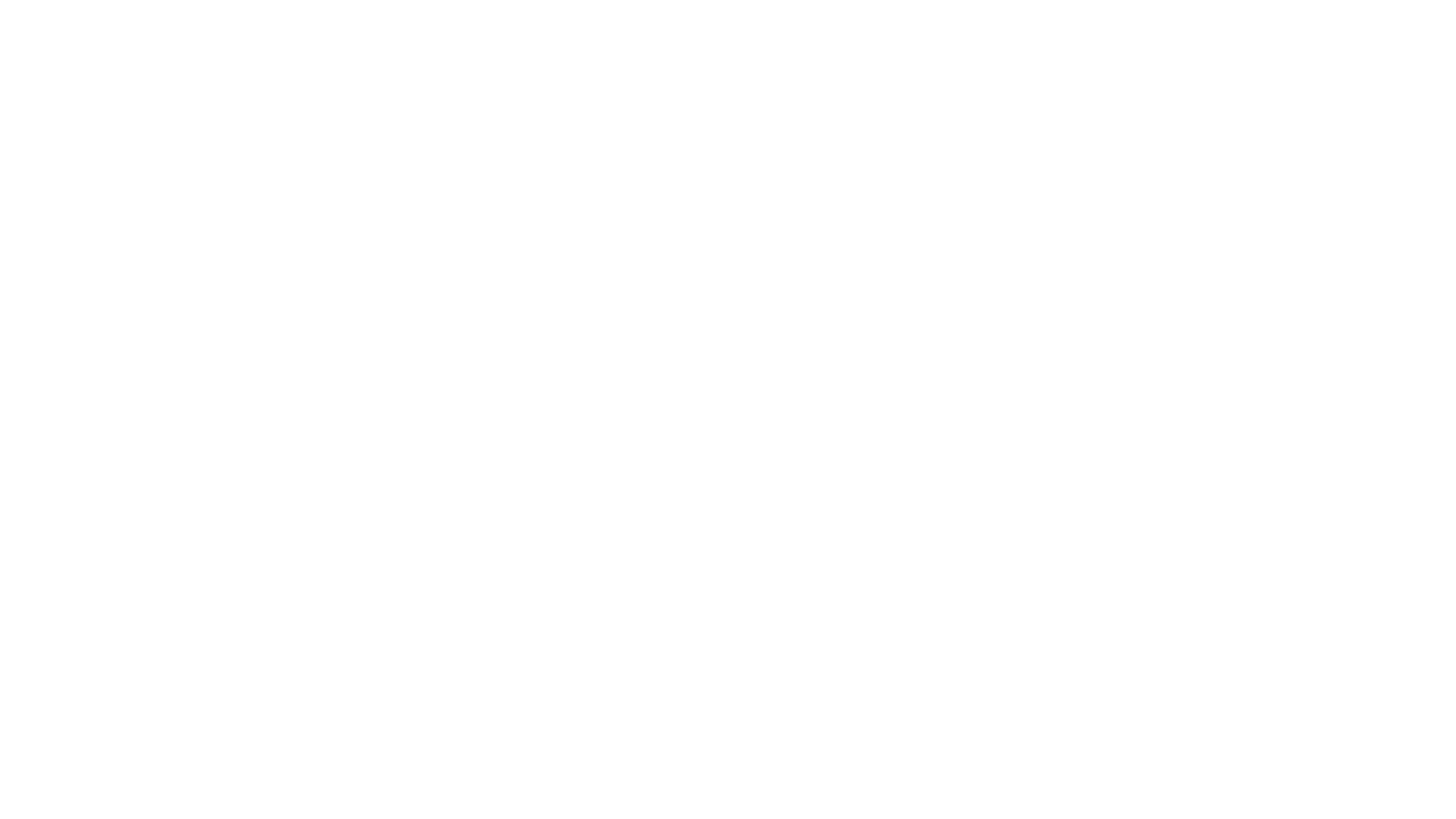
COMPUTER SCIENCE AND ENGINEERING
BIOLOGICAL SCIENCES AND BIOENGINEERING
Cell Lineage Inference
Reconstructing cell lineages that lead to the formation of tissues and organs is of crucial importance in developmental biology. Very recently, experimental methods are starting to combine two novel technologies, single-cell RNA-sequencing and CRISPR-Cas9 barcode editing for elucidating developmental lineages at the whole organism level. scGESTALT and ScarTrace are two such methods that have been applied for studying the development in Zebrafish. scGESTALT combines the CRISPR-Cas9-based lineage tracing method GESTALT with droplet-based single-cell transcriptomic profiling. ScarTrace utilizes identical target sites located on separate transgenes for introducing CRISPR-Cas9 mutations followed by SORT-seq sequencing to capture the transcriptome. However, these studies resorted to using off-the-shelf tree reconstruction algorithm such as maximum parsimony for reconstructing the lineage tree based on the stochastic Cas9-induced mutations. Such an approach has several limitations arising from the noisy and often saturated random mutation data. Lineage trees reconstructed solely based on mutation data sometimes fails to separate different types of cells and places similar cell types on distant branches. Additionally, due to the randomness of the mutations, lineages from multiple experiments cannot be combined to reconstruct a consensus lineage tree. To address these issues, we are developing novel statistical tree inference algorithms that can integrate mutation and transcriptomic data under a probabilistic framework and overcome mutational noise with the help of transcriptomic data. In addition, we are also developing computational models for reconstructing consensus lineages that can combine data from multiple individuals studied using methods such as scGESTALT or ScarTrace. The figure below gives an overview of our lineage reconstruction methods.
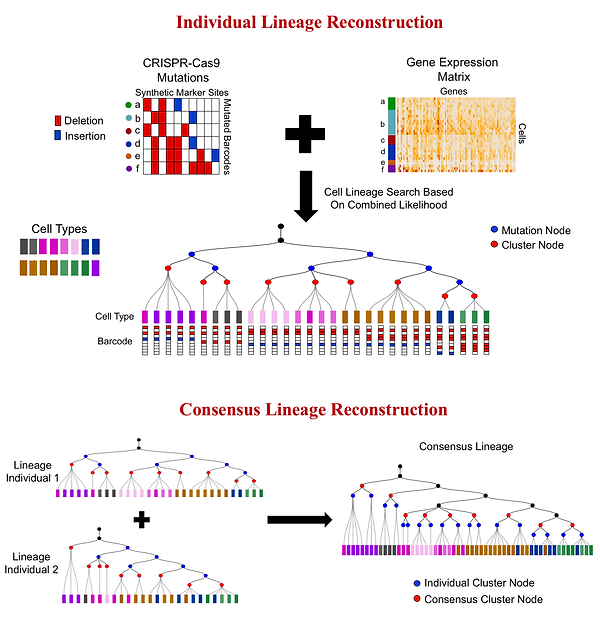