
COMPUTER SCIENCE AND ENGINEERING
BIOLOGICAL SCIENCES AND BIOENGINEERING
Elucidating Tumor Heterogeneity and Evolution
Cancer is a disease driven by a complex interplay of somatic mutations in a Darwinian framework of fitness and selection that results in a mixture of multiple genotypically and phenotypically distinct cell populations. From a clinical perspective, this intratumor heterogeneity complicates the diagnosis and treatment of cancer patients and causes relapse and drug resistance. Previously, we developed SiFit and SiCloneFit for inferring tumor phylogenies from single-cell sequencing (SCS) data, which provides the highest resolution into tumor heterogeneity. However, due to higher cost and technical errors, SCS is still not the primary approach in studying cancer. Till date, most cancer studies resorted to bulk high-throughput sequencing. The admixture signal in variant allele frequencies (VAFs) of mutations detected from bulk sequencing necessitates deconvolution of the subpopulations. Such datasets provide a global picture of tumor heterogeneity. However, bulk sequencing and single-cell sequencing provide complementary knowledge and if leveraged in a principled manner can improve inference of clonal composition and abundance over a standalone approach.
We are interested in developing a unified computational framework for the joint analysis of bulk and single-cell sequencing datasets. Usually, in single-cell sequencing studies, bulk tumor and normal tissue are also sequenced to serve as the repository of the global knowledge. Simultaneous integration of mutation profiles from both bulk tissue and single cells in a single probabilistic framework can improve the inference of clonal composition, genotypes and phylogeny. The inclusion of knowledge from bulk data can also improve variant calling in single cells to distinguish rare variants from technical artifacts. The figure below outlines the proposed unified framework.
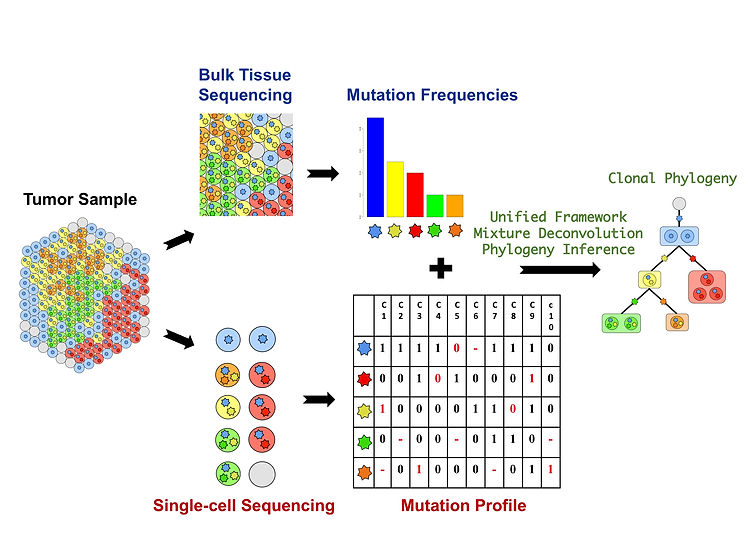